Why Conventional Product Testing Gets Flavor So Wrong
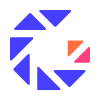
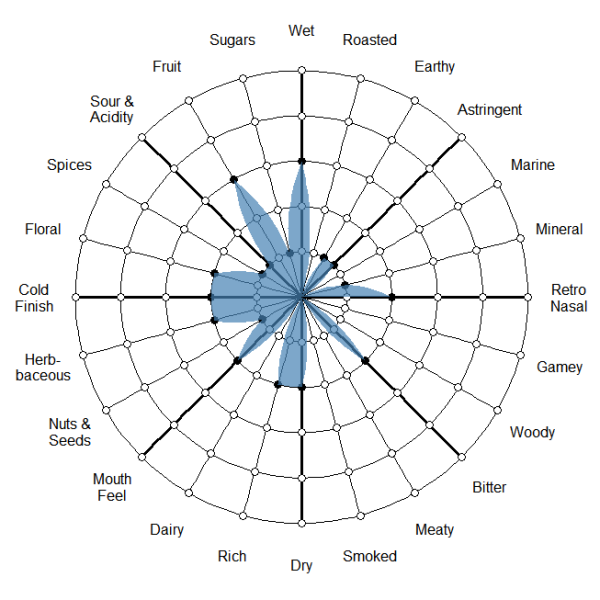
Can you remember the best meal you ever tasted?
For me, it was a mind-blowing seafood pasta during a holiday in Rome. I could tell you that the prawns were juicy, the sauce was tangy, and the pasta had a great texture.
But I’d struggle to describe it further.
The dish was just…wonderful. Delicious. Perfect.
The thing is, it’s pretty hard to accurately put words to flavor. And food that we find truly great or truly terrible often leaves us speechless.
Describing flavors in a way that’s useful for product development is even trickier — because flavors are complicated, and taste is a subjective experience.
Conventional sensory analysis and product testing don’t give you accurate and reliable results because they can’t account for the complexity of flavor and the tasting experience. Gastrograph AI can.
How Does Conventional Product Development Deal With Flavor?
In conventional product testing, reference-trained experts taste products and distill them into a list of flavor attributes.
CPG companies typically use Central Location Tests (CLTs) to test their products. First, expert tasters do descriptive analyses: they create a list of the flavor attributes that are present in the product. The list is a consensus, so if only one or two members of the panel taste a flavor, it doesn’t make it onto the list.
Then, consumers do preference analyses. They rate how much they like the attributes on the list and their overall preference for the product.
What’s Wrong With That Approach?
The conventional process of generating an attributes list by consensus oversimplifies what happens when we taste a new product. It also doesn’t account for the fact that taste is subjective and language is messy.
Taste Buds Aren’t Consistent
We might taste the same product and perceive it differently. Take cilantro: to some, it’s a delicious, fresh, citrus flavor. By limiting the attributes list to only those the consensus agrees on, you don’t get a full flavor profile, and you might miss tasting data (i.e., the fact that your product tastes like soap to some people).
Language is Tricky
Even if we do perceive the same flavors, we might disagree on the best way to describe them. Imagine we’re both eating fresh chocolate eclairs in a French patisserie shop. We perceive the pastry in the same way (flipping delicious). But you describe it as “rich,” and I describe it as “creamy.”
Based on our life experiences, we’ll interpret terms differently. Let’s say we leave the pastry shop and pick up some licorice sticks. Again, let’s imagine we perceive the flavor in the same way. You say the licorice tastes like anise. But I’ve never heard of anise, so I describe the licorice as “uuhh...kind of...minty?”
Expert Tasters Don’t Represent Consumers
The expert tasters used for the perception round of CLTs are highly skilled. They’ve gone through extensive training, which means they’re able to taste products and identify the flavors in relation to references they’ve learned. But they don’t represent normal consumers, so you’re warping your perception data.
Perception Affects Preference
When you have a separate round for perception analysis with experts and one for preference analysis with consumers, you separate perception from preference. You limit your ability to understand what attributes make people like or dislike your product. You also risk getting dodgy data because of flavor offloading.
How Gastrograph Treats Flavor (And Why It’s Better Than Conventional Methods)
Conventional testing tries to squash the tasting experience to fit into a simple system that’s easy to analyze. Instead, we’ve designed our system so it's able to capture rich tasting data and understand flavor in its full complexity.
We Respect the Individual Tasting Experience
When we bring new products onto the Gastrograph system, individual tasters do all the flavor perception, intensity, and preference analysis. Our perception and preference data are connected. You’re able to identify which flavor attributes drive liking and which have a negative impact on preference.
Plus, the tasters have spent time thinking about the flavors in a product to fill out the flavor profile before they rate their preference. So preference scores are more accurate than if tasters were immediately asked to rate a product without creating the profile.
In conventional testing, consumer tasters are told by the experts which attributes are present — so they’re primed to taste certain flavors. In comparison, people who taste for Gastrograph are always asked to add flavor labels under the same 24 attributes. Our method means we get a truer, less biased picture of what people actually taste.
We Allow For the Messiness of Language
With Gastrograph AI, people can describe products in a way that makes sense to them. Our system uses Natural Language Processing to analyze and understand their flavor descriptions. It’s just like how Google Search interprets what you type into the search bar to give you the results you’re looking for.
In the Gastrograph system, there are 24 flavor attributes that cover all the different types of flavors someone could experience in the course of a normal tasting.
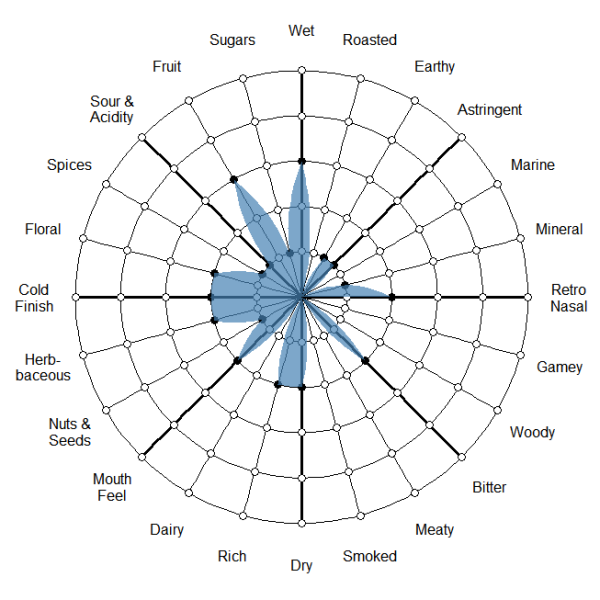
Tasters can add as many flavor labels as they want under these 24 attributes. They can also use whatever words they want to describe the flavor in whatever language they want. Jason Cohen, CEO and founder of Gastrograph AI, explains, “there's no constraint on the lexicon that they can use. Some people are like, well, I tasted this thing, and I only know the Chinese phrase, and they type it in Chinese. Totally okay.”
We Understand the Complexity of Flavor
Gastrograph’s layered flavor signatures capture the complexity of flavor. Instead of having one flavor linked to one word, we’ve trained our system to understand flavors as layered signatures.
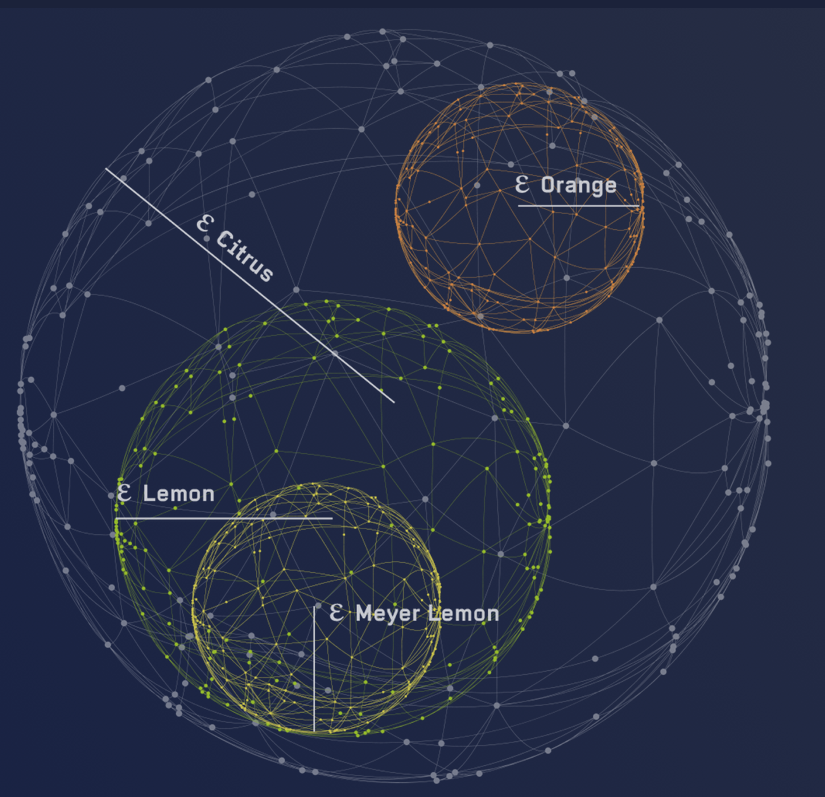
The terms within flavors are hierarchical, and they overlap. For example, Meyer lemon is a subflavor of lemon, which is a subflavor of citrus. The layering of flavors means that tasters can describe flavor differently, and the system will understand what they’re describing.
It’s similar to how predictive text works. You type in a few key parts of a word or phrase, and your phone knows what you intend to write — even if you spell something wrong. One taster might describe something as “peppery,” and another could describe it as “spicy.” The Gastrograph system will analyze these descriptive labels, understand the intended meaning, and identify if they’re part of the same flavor signature.
We also use flavor maps instead of attribute lists, so we’re able to visualize the balance of flavors more easily within a product.
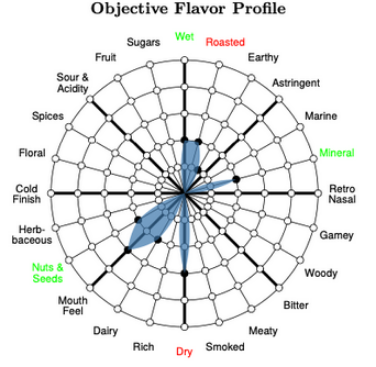
The maps (objective flavor profiles) convey the flavors people perceive and at what intensity, represented by the blue area on the map. The further the blue extends from the center, the more intensely someone perceived that flavor. The colors also communicate the impact of the flavors on preference: green is positive, red is negative.
In the past, it was necessary to simplify flavor because it was too time-consuming to analyze and draw meaningful insights from complex tasting data. Today there’s no excuse. The power of AI means our system can process a lot of information in a short time. We can gather nuanced and detailed tasting data and use our system to spot patterns and make predictions.
Bring Magic to Your Product Development
Imagine if you could understand consumers so intricately that you knew exactly what kind of products to make to delight them. Gastrograph helps you navigate the wonderfully complex world of flavor. You’ll be able to create products so amazing that people will want to write poetry.
Featured Articles

Leveraging AI to achieve super-human performance in Food & Beverage product optimization across cultures
Pangborn 2023 Poster
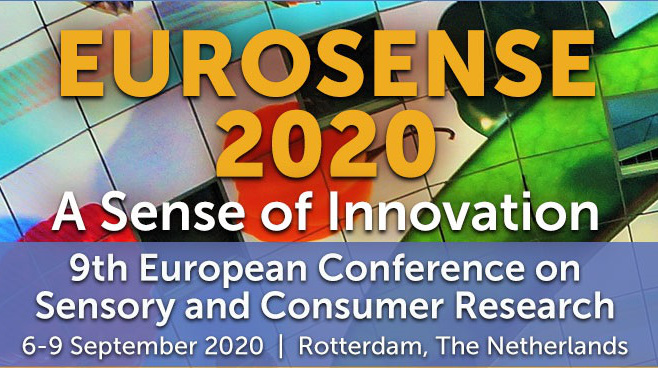
Consumer Preference Changes induced by COVID-19 quarantine for the General Population United States
COVID-19 induced consumer preference trends identified by predictive sensory analysis
Read our Whitepaper
Our double-blind validation study shows how Gastrograph AI outperforms traditional product testing.